BERT1Bidirectional Encoder Representations (and it’s numerous variants) models have taken the natural language processing field by storm ever since they came out and have been used to establish state of the art results in pretty much all imaginable tasks, including text analysis.
I am a Christian, so the Bible is important to me. So, I became curious to see what BERT would “think” about the Bible. The manuscripts of the Bible on which modern translations are based were written in Hebrew (Old Testament) and Greek (New Testament). There are many difficult challenges in the translation, resulting in many debates about the meaning of some words. I will conduct two experiments on the text of the New Testament to see what BERT outputs about the various forms of “love” and about the distinction between “soul” and “spirit”.
Quick BERT primer
There are many good explanations of how BERT works and how it’s trained, so I won’t go into that, I just want to highlight two facts about it:
- one of the main tasks that is used to train a BERT model is to predict a word2actually a byte pair encoded token given it’s context: “Today is a [MASK] day”. In this case it would have to predict the fourth word and possible options are “beautiful”, “rainy”, “sad” and so on.
- one of the things that BERT does really well is to create contextual word embeddings. Word embeddings are mathematical representations of words, more precisely they are high dimensional vectors (768 in the case of BERT), that have a sort of semantic meaning. What this means is that the word embedding similar words is close to each other, for example, the embeddings for “king”, “queen” and “prince” would be close to each other, because they are all related to royalty, even though they have no common lemma. The contextual part means there is no one fixed word embedding for a given word (such as older models like word2vec or GloVe had), but it depends on the sentence where the word is used, so the word embedding for “bank” is different in the sentence “I am going to the bank to deposit some money” than in the sentence “He is sitting on the river bank fishing”, because they refer to different concepts (financial institution versus piece of land).
Obtaining the embeddings
Reading the data
First, let’s read the Bible in Python. I’ve used the American King James Version translation, because it uses modern words and it’s available in an easy to parse text file, where the verse number (Matthew 15:1) is separated from the text of the verse by a tab (\t
):
Genesis 1:1 In the beginning God created the heaven and the earth.
verses = {}
with open('akjv.txt', 'r', encoding='utf8') as f:
lines = f.readlines()
for line in lines[23146:]: # The New Testament starts at line 23146
citation, raw_sentence = line.strip().split('\t')
verses[citation] = raw_sentence
The next thing we need is the Strong’s numbers, which are a code for each Greek word (or rather base lemma) that appears in the New Testament. I have found a mapping to tell me the corresponding Strong’s number for (most) English words only for the ESV3I had to rename the New Testament book names and convert the file to UTF8 without BOM translation, which might mean that there are slight differences in verse boundaries, but I don’t think that the words I’m going to be analyzing will be different. Here the format is also verse number, followed by xx=<yyyy>
pairs, where xx is the ordinal number for a word in the ESV translation and yyyy is the corresponding Strong’s number.
Matthew 1:1 02=<0976> 05=<1078> 07=<2424> 08=<5547> 10=<5207> 12=<1138> 14=<5207> 16=<0011>
This line says that in Matthew 1:1 the second word in the ESV translation corresponds to the Greek word with Strong’s number 976, the fifth word in English to the word with Strong’s number 1078 and so on. The Strong’s numbers are nicely preformatted into 4 character strings, so we check if a Strong’s number is in a verse by simply looking if the number is in this string, without having to parse each verse.
strongs_tags = {}
with open("esv_tags.txt") as f:
lines = f.readlines()
for line in lines:
verse, strongs = line.split("\t", maxsplit=1)
strongs_tags[verse] = strongs
Getting the embedding for a word with BERT
Let’s load the BERT model and it’s corresponding tokenizer, using the HuggingFace library:
from transformers import AutoTokenizer, AutoModel
tokenizer = AutoTokenizer.from_pretrained('bert-base-cased')
model = model = AutoModel.from_pretrained('bert-base-cased', output_hidden_states=True).eval()
BERT has a separate tokenizer because it doesn’t work on characters or on words directly, but it works on byte pair encoded tokens. For more frequent words, there is a 1:1 mapping of word – token, but rarer words (or words with typos) will be split up into multiple tokens. Let’s see this for the word “love” and for “aardvark”:
encoded = tokenizer.encode_plus("I love pizza", return_tensors="pt")
print(encoded.tokens())
> ['[CLS]', 'I', 'love', 'pizza', '[SEP]']
print(encoded.word_ids())
> [None, 0, 1, 2, None]
[CLS]
and [SEP]
are two special tokens, mostly relevant during training. The word_ids
function returns the index of the word to which that token belongs. Let’s see an example with a rare word:
> encoded = tokenizer.encode_plus("I love aardvarks", return_tensors="pt")
encoded.tokens()
> ['[CLS]', 'I', 'love', 'a', '##ard', '##var', '##ks', '[SEP]']
print(encoded.word_ids())
> [None, 0, 1, 2, 2, 2, 2, None]
In this case, aardvarks (the second word) is split up into 4 tokens, which is why it shows up 4 times in the list obtained from word_ids
.
Now, let’s find the index of the word we are looking for in a verse:
def get_word_idx(sent: str, word: str):
l = re.split('([ .,!?:;""()\'-])', sent)
l = [x for x in l if x != " " and x != ""]
return l.index(word)
We split on punctuation and spaces, skip empty strings and ones with a space and get the index of the word we are looking for.
Because the BPE encoding can give multiple tokens for one word, we have to get all the tokens that correspond to it:
encoded = tokenizer.encode_plus(sent, return_tensors="pt")
idx = get_word_idx(sent, word)
# get all token idxs that belong to the word of interest
token_ids_word = np.where(np.array(encoded.word_ids()) == idx)
In BERT, the best word embeddings have been obtained by taking the sum of the last 4 layers. We pass the encoded sentence through the model to get the outputs at the last 4 ones, sum them up layerwise and then average the outputs corresponding to the tokens that are part of our word:
def get_embedding(tokenizer, model, sent, word, layers=None):
layers = [-4, -3, -2, -1] if layers is None else layers
encoded = tokenizer.encode_plus(sent, return_tensors="pt")
idx = get_word_idx(sent, word)
# get all token idxs that belong to the word of interest
token_ids_word = np.where(np.array(encoded.word_ids()) == idx)
with torch.no_grad():
output = model(**encoded)
# Get all hidden states
states = output.hidden_states
# Stack and sum all requested layers
output = torch.stack([states[i] for i in layers]).sum(0).squeeze()
# Only select the tokens that constitute the requested word
word_tokens_output = output[token_ids_word]
return word_tokens_output.mean(dim=0)
Processing the New Testament with BERT
Now let’s get the embeddings for the target words from all the verses of the New Testament. We will go through all the verses and if any of the Strong’s numbers appear in the verse, we will start looking for a variation of the target word in English and get the embedding for it. The embedding, the verse text, the Greek word and the book where it appears will be appended to a list.
def get_all_embeddings(greek_words, english_words):
embeddings = []
for key, t in verses.items():
strongs = strongs_tags[key]
for word in greek_words:
for number in greek_words[word]:
if number in strongs:
gw = word
for v in english_words:
try:
if v in t:
emb = get_embedding(tokenizer, model, t, v).numpy()
book = books.index(key[:key.index(" ", 4)])
embeddings.append((emb, f"{key} {t}", gw, book))
break
except ValueError as e:
print("Embedding not found", t)
else:
print("English word not found", key, t)
return embeddings
Next, I am going to take all the verses where one of these target words appear in the New Testament. I am going to mask out their appearance and ask BERT to predict what word should be there.
def mask_and_predict(word_list):
predictions = []
for key, t in verses.items():
for v in word_list:
if v in t:
try:
new_t = re.sub(f"\\b{v}\\b", "[MASK]", t)
top_preds = unmasker(new_t)
if type(top_preds[0]) == list:
top_preds = top_preds[0]
predictions.append((f"{key} {t}", v, top_preds))
break
except Exception:
print(new_t, v)
return predictions
Love
In Greek, there are several words that are commonly translated as love: agape, eros, philia, storge, philautia, xenia, each having a different focus/source. In the New Testament, two of these are used: agape and philia. There is much debate between Christians about the exact meaning of these two words, such as whether agape is bigger than philia, the two are mostly synonyms, or philia is the bigger love.
To try to understand what BERT thinks about these two variants, I am going to extract the 768 dimensional word embeddings for the English word love, reduce their dimensionality with UMAP and plot the results, color coding them by the original word used in Greek.
Now we’ll need the Strong’s numbers for the two words we’re investigating. I included several variations for each word, such as verbs/nouns, or composite variants, such as 5365 – philarguria, which is philos + arguria, meaning love of money.
strongs_numbers = {
"agape": ["0025", "0026"],
"phileo": ["5368", "5360", "5363", "5362", "5361", "5366", "5365", "5377"]
}
word_list = ["lovers", "loved", "loves", "love", "Love"]
embeddings = get_all_embeddings(strongs_numbers, word_list)
There are some weird failure cases: in 1 Corinthians 13, famously called the chapter of love, the AKJV uses charity for example instead of love for the Greek word “agape”. I chose to not look for charity as well, so all those uses of “agape” are left out.
Now that we have all the embeddings, let’s reduce their dimensionality with UMAP and then visualize them. They will be color coded according to the Greek word and on hover they will show the verse.
The blue dots are where the Greek is agape (or it’s derivatives), while the red ones are where the Greek is philos.
You can notice 4 clusters in the data. The top right cluster is mostly made out of love that is between Christians. The bottom right one seems to be mostly about the love of God, with the love of money throw in there as well (the blue dot on the right). The cluster on the left seems to be less well defined, with the top side looking like it’s about commandments related to love (you shall love, should love, if anyone will love) and it’s consequences. The bottom left side is the most fuzzy, but I think it seems to be about the practical love of Jesus for humans.
What is easier to notice is that the Greek words agape and philos are mixed together. The love of God cluster (bottom right) seems to be the only one that is agape only (if we exclude the love of money verse, which reeaaaally doesn’t belong with the others), with the exception of the Titus 3:4 verse, which however does sound very much like the others.
However, we can plot the same graph, but this time color coding with the parts of the New Testament where the verse is found:
There is lots of mixing in all clusters, but it seems to me that the Pauline letters use love in a different way then the gospels.
Conclusion? Yes, the word agape does sometimes refer to the love of God, in a seemingly special way, but it often refers to other kinds of love as well, in a way which BERT can’t really distinguish from philos love.
Soul and spirit
The Bible uses two words for the immaterial parts of man: soul (Hebrew: nephesh, Greek: psuche) and spirit (Hebrew: ruach, Greek: pneuma). Again, there is great debate whether the two are used interchangeably or whether they are two distinct components of humans.
strongs_numbers = {
"pneuma": ["4151"],
"psuche": ["5590"],
}
word_list = ["spirits", "souls", "Spirits", "soul", "spirit","Spirit"]
embeddings = get_all_embeddings(strongs_numbers, word_list)
After getting the embeddings for these two words, I will plot them as we did before. We can discover quite a few clusters in this way.
In this case, the clusters are almost perfectly separated, with very little mixing. What little mixing happens is usually because in one verse both words occur. Contrast this with the case for agape/phileo, where there is a lot of mixing.
The top right cluster is about the Spirit of God. The one below is about unclean/evil spirits. The middle cluster is about the Holy Spirit. The bottom left cluster is mostly about the spirit of man, with some examples from the other clusters.
The interesting thing is that the two verses used as most common arguments for the soul being distinct from the spirit (1 Thessalonians 5:23 and Hebrews 4:12) are placed in the blue cluster, and they are right next to Matthew 22:37, Mark 12:30 and Luke 10:27, verses which indicate that man is made of different components (heart, soul, mind, strength).
Now, let’s mask out the words soul and spirit and ask BERT to predict the missing word. If BERT mixes the two half the time, it means it thinks there is no distinction between them. Otherwise, they are probably distinct. The resulting confusion matrix:
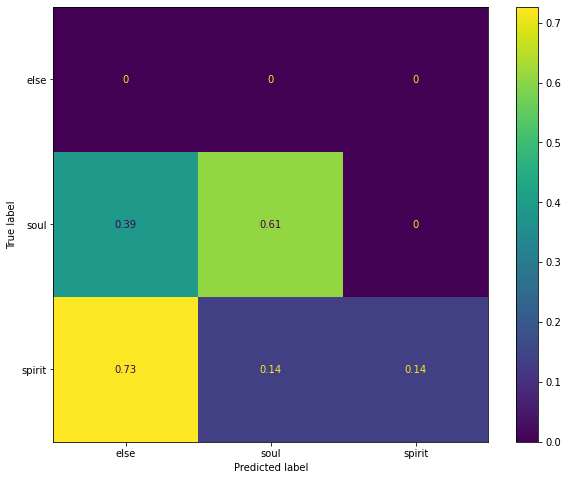
The y axis represent the true word (soul or spirit), the x axis represents the predicted word (something else, soul or spirit). We can see that in more that 60% of the cases it predicts soul correctly. It never mispredicts it to spirit, but in 40% of the cases it does predict something else. For spirit, the results are worse: it predicts something else quite often, and on top of that, it predicts 50-50% between soul and spirit, so it mixes them up quite often.
The conclusion? The evidence is mixed: on one hand, usages of soul and spirit seem to be mostly different, because they cluster very neatly. But some key verses for the distinction are put in the soul cluster. Now, this might happen because of the way BERT extracts embeddings, two words that are in the same sentence will have similar embeddings. On the other hand, because of the way spirit is mispredicted, it would seem to indicate that there is significant overlap between spirit and soul, at least as “understood” by BERT.
Conclusion
I believe with some polish, BERT-style models can eventually make their way into the toolbox of someone who studies the Bible. They can offer a more consistent perspective to analyzing the text. And of course, they can be used not just to analyze the Bible, but for many other purposes, such as building tools for thoughts (using computers to help us think better and faster), or to analyze all kinds of documents, to cluster them, to extract information from them or to categorize them.
If you need help with that, feel free to reach out to me.
The full code for this analysis can be found in this Colab.