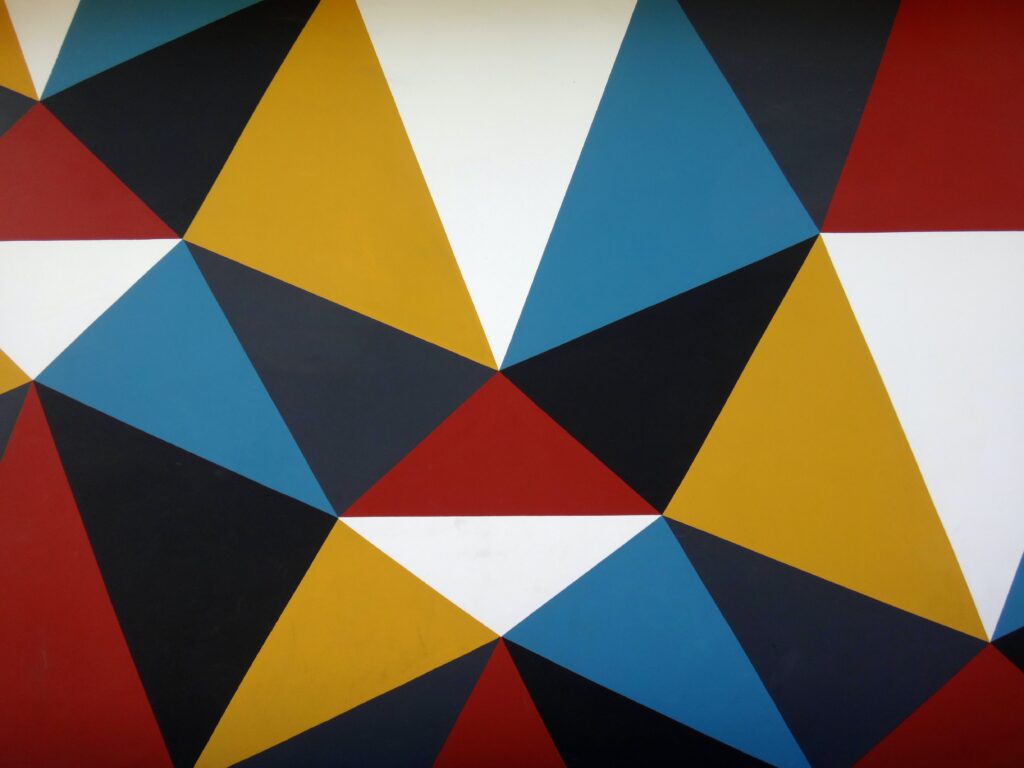
Prodigy is a great tool for annotating the datasets needed to train machine learning models. It has built in support for many kinds of tasks, from text classification, to named entity recognition and even for image and audio annotation.
One of the cool things about Prodigy is that it integrates with Spacy (they are created by the same company), so you can use active learning (having a model suggest annotations and then being corrected by humans) or you can leverage Spacy patterns to automatically suggest annotations.
Prodigy has various recipes for these things, but it doesn’t come with a recipe to use only patterns for manual annotation for a multilabel text classification problem, only in combination with an active learning loop. The problem is that for multi-label annotation, Prodigy does binary annotation for each document, meaning the human annotator will be shown only one label at a time and they’ll have to decide if it’s relevant to the document or not. If you have many labels, it means each document might be shown as many times as there are labels.
I recently had to solve a problem where I knew that most of the documents would have a single label, but in a few cases there would be multiple labels. I also had some pretty good patterns to help bootstrap the process, so I wrote a custom recipe that used only patterns for a multilabel text classification problem.
Code for custom recipe
To do this, I combined some code from the recipes that are provided by Prodigy for text categorization. Let’s see how it work.
First, let’s define the CLI arguments in a file called manual_patterns.py
. We’ll need:
@recipe(
"textcat.manual_patterns", # Name of the recipe
dataset=("Dataset to save annotations to", "positional", None, str),
source=("File path with data to annotate", "positional", None, str),
spacy_model=("Loadable spaCy pipeline or blank:lang (e.g. blank:en)", "positional", None, str),
labels=("Comma-separated label(s) to annotate or text file with one label per line", "option", "l", get_labels),
patterns=("Path to match patterns file", "option", "pt", str),
)
Then we need to define the function that loads the stream of data, runs the PhraseMatcher on it and returns the project config:
def manual(
dataset: str,
source: Union[str, Iterable[dict]],
spacy_model: str,
labels: Optional[List[str]] = None,
patterns: Optional[str] = None,
):
log("RECIPE: Starting recipe textcat.manual_patterns", locals())
log(f"RECIPE: Annotating with {len(labels)} labels", labels)
stream = get_stream(
source, rehash=True, dedup=True, input_key="text"
)
nlp = spacy.load(spacy_model)
matcher = PatternMatcher(nlp, prior_correct=5.0, prior_incorrect=5.0,
label_span=False, label_task=True, filter_labels=labels,
combine_matches=True, task_hash_keys=("label",),
)
matcher = matcher.from_disk(patterns)
stream = add_suggestions(stream, matcher, labels)
return {
"view_id": "choice",
"dataset": dataset,
"stream": stream,
"config": {
"labels": labels,
"choice_style": "multiple",
"choice_auto_accept": False,
"exclude_by": "task",
"auto_count_stream": True,
},
}
The last bit is the function which takes the suggestions generated by the PhraseMatcher and adds them to be selected by default in the UI. In this way, the annotators can quickly accept them:
def add_suggestions(stream, matcher, labels):
texts = (eg for score, eg in matcher(stream))
options = [{"id": label, "text": label} for label in labels]
for eg in texts:
task = copy.deepcopy(eg)
task["options"] = options
if 'label' in task:
task["accept"] = [task['label']]
del task['label']
yield task
Expected file formats
Now let’s run the recipe. Assuming we have an news_headlines.jsonl
file in the following format:
{"text":"Pearl Automation, Founded by Apple Veterans, Shuts Down"}
{"text":"Silicon Valley Investors Flexed Their Muscles in Uber Fight"}
{"text":"Uber is a Creature of an Industry Struggling to Grow Up"}
{"text": "Brad Pitt is divorcing Angelina Jolie"}
{"text": "Physicists discover new exotic particle"}
And an pattern file patterns.jsonl
:
{"pattern": "Uber", "label": "Technology"}
{"pattern": "Brad Pitt", "label": "Entertainment"}
{"pattern": "Angelina Jolie", "label": "Entertainment"}
{"pattern": "physicists", "label": "Science"}
Running the custom recipe
You can start Prodigy with the following command:
> python -m prodigy textcat.manual_patterns news_headlines news_headlines.jsonl blank:en --label "Science,Technology,Entertainment,Politics" --patterns patterns.jsonl -F .\manual_patterns.py
Using 4 label(s): Science, Technology, Entertainment, Politics
Added dataset news_headlines to database SQLite.
D:\Work\staa\prodigy_models\manual_patterns.py:67: UserWarning: [W036] The component 'matcher' does not have any patterns defined.
texts = (eg for score, eg in matcher(stream))
✨ Starting the web server at http://localhost:8080 ...
Open the app in your browser and start annotating!
And you should see the following in the browser:
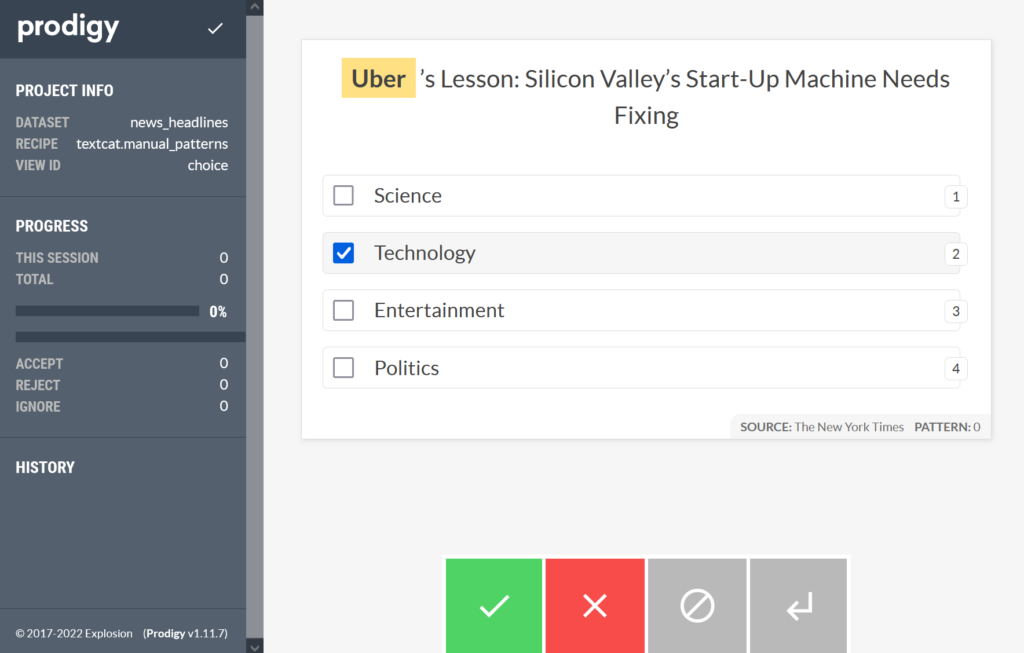
The full code for the recipe can be found here.